Fine-Tuning Your LLM? There’s a Better Way
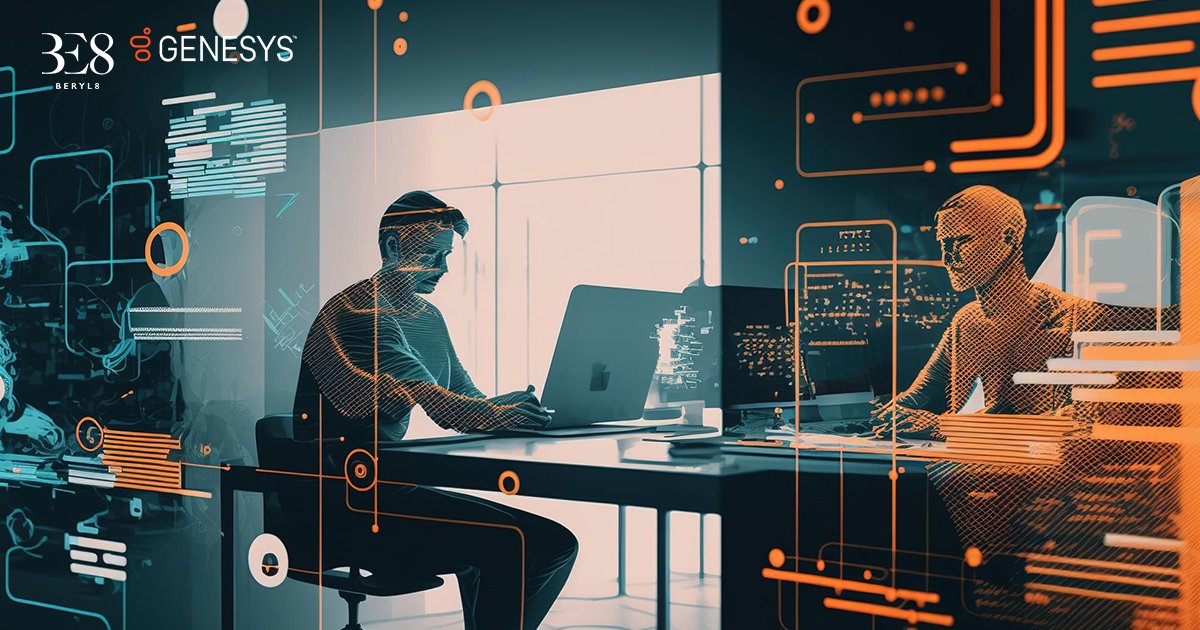
The next leap in AI fine-tuning may be no fine-tuning at all. Vector databases make it easier for you to access and analyze all your business data.
Ever get an email trying to sell you a product you’ve already bought? Or had a service interaction where you had to answer the same questions multiple times with different people?
Large language models (LLMs) promise to eliminate these annoyances by providing greater levels of information-sharing and personalization within your company’s operations. The problem is, off-the-shelf LLMs, such as OpenAI’s ChatGPT and Google’s Bard, which are used by many companies, are built with generic data universally available on the internet. Since they don’t have access to your proprietary data, any AI that’s built on top of them won’t deliver the nuance your customers expect. And generic data isn’t always up to date. ChatGPT’s data only goes up to January 2022, for example.
To tailor off-the-shelf LLMs to your company’s needs, you’ll have to incorporate your own company data into the artificial intelligence (AI) model. This process, called fine-tuning, may yield better results for your customers. But it’s expensive and time-consuming, and it might raise trust issues.
There’s a better way: A vector database, “a new kind of database for the AI era” that offers all the benefits of fine-tuning, and also alleviates privacy concerns, helps unify data, and saves time and money.
What is LLM fine-tuning?
Fine-tuning an LLM means training it to make it better at specific tasks, like analyzing customer sentiment or summarizing a patient’s health history.
With fine-tuning, you expose the model to examples or data related to the task you want it to complete. For instance, a law firm might fine-tune an LLM with information about legal clauses and terms to train it to extract certain information from documents.
But fine-tuning is costly, requiring lots of compute power, specific expertise, and additional infrastructure. And it’s time-consuming because large models require lots of time to train. The larger the model, the more time needed.
Further, fine-tuning is merely a stopgap that fails to address a more fundamental shortfall: the lack of unified data. Why should you care about unified data? Because when your company data is siloed in different parts of your organization, your customers get a disjointed, repetitive experience.
“Fine-tuning is still an unknown, and the benefits are unproven,” said Rahul Auradkar, EVP of product management at Salesforce. “If you fine-tune models using data that is pertinent to your customers, you are injecting some of their data into the model, which really raises a lot of trust issues.”
Enter stage left: the vector database
A vector database can either plug directly into an LLM or the prompt. It’s called a vector database because it organizes and stores data in a way that emphasizes vectors, which are tags that describe different types of data in detail. These descriptors help you find relevant information in a sea of data, regardless of its origin.
For example, companies managing large supply chains can use a vector database to analyze and optimize shipping routes. The vector database can store information about traffic patterns, weather conditions, and road closures. Or, an AI chatbot on a self-service page will know if a customer is eligible for an upgrade or special offer because it’s synthesizing relevant data from the right sources at the right time.
In this way, a vector database eliminates the need for fine-tuning, and unifies all your enterprise data with your CRM in one fell swoop.
This is critical for the accuracy, completeness, and efficiency of the outputs, or answers, you get from AI prompts. Here’s why: The vast majority (90%) of corporate data lives in so-called unstructured formats like PDFs, text documents, video, email, and social media posts, making it largely inaccessible to business apps and AI models. Because it lacks a structured, organized format, it’s almost impossible for LLMs to analyze.
“Unstructured data is super valuable to companies, but it’s very hard to act upon,” said Auradkar, “Companies want to bring this unstructured data to life.”
Your proprietary data is a gold mine – use it
A company’ proprietary data is the foundation for building an enterprise LLM. A vector database lets AI store and process all this data in a way that’s easy to understand and analyze.
This increases business value and ROI How? It combines unstructured data and structured data, including purchase history, customer support cases, and product inventory, to power AI, automation, and analytics across every business application. When you have access to all this information, you can make better decisions that result in better business outcomes.
If you are interested in more information about Salesforce, contact our team of experts by clicking.